Before:
Siloed Fan Data Impacted Business Growth Opportunities
The Giants had hordes of avid fans and swaths of fan data. The challenge was putting it all together to gain insight. Their existing data and analytics solution presented several challenges.
First, people who needed the data couldn’t access it. All the data was siloed, and the company lacked a single, centralized source of truth. Second, the data was untrustworthy, containing duplicate records that yielded incomplete or incorrect information.
All of this slowed down reporting and made the team hesitant to even start analysis. Knowing a change was needed, they turned to Data Clymer, a Spaulding Ridge company, for the solution.
Solution:
Unlocking Fan Insights Through a Master Data Management Solution
After reviewing with the Giants, we decided to implement Snowflake and a master data management solution that put timely, accurate insights into the hands of those who need them.
Striving for democratized data for their sales and marketing teams, we implemented a modern cloud data platform comprised of Snowflake, Matillion, Melissa Data, and Tableau.
With native support of JSON files, the data team could store results from Melissa, their new master data management platform, as a variant data type directly in Snowflake. Then they could easily flatten the data into a structured format for further transformation processing.
As a cloud-native ELT tool, Matillion was the product of choice to bring data from multiple siloed sources into Snowflake and transform it into an analytics-ready format. Matillion is designed to work with Snowflake, enabling the team to:
- Leverage the power of Snowflake to handle large volumes of data quickly and at scale.
- Dedicate Snowflake compute power to Matillion jobs so the team can run Matillion jobs and analytical queries at the same time.
- Create custom metadata-driven ELT applications by creating configuration tables in Snowflake. This allows the team to extract a new table from a source without touching the Matillion code; they just need to add a new entry into a configuration table.
Melissa enabled the teams to clean email addresses and mailing addresses leveraging access to the US Postal Service address database (among other libraries). The Giants could identify if an address is business or residential and attach a latitude and longitude.
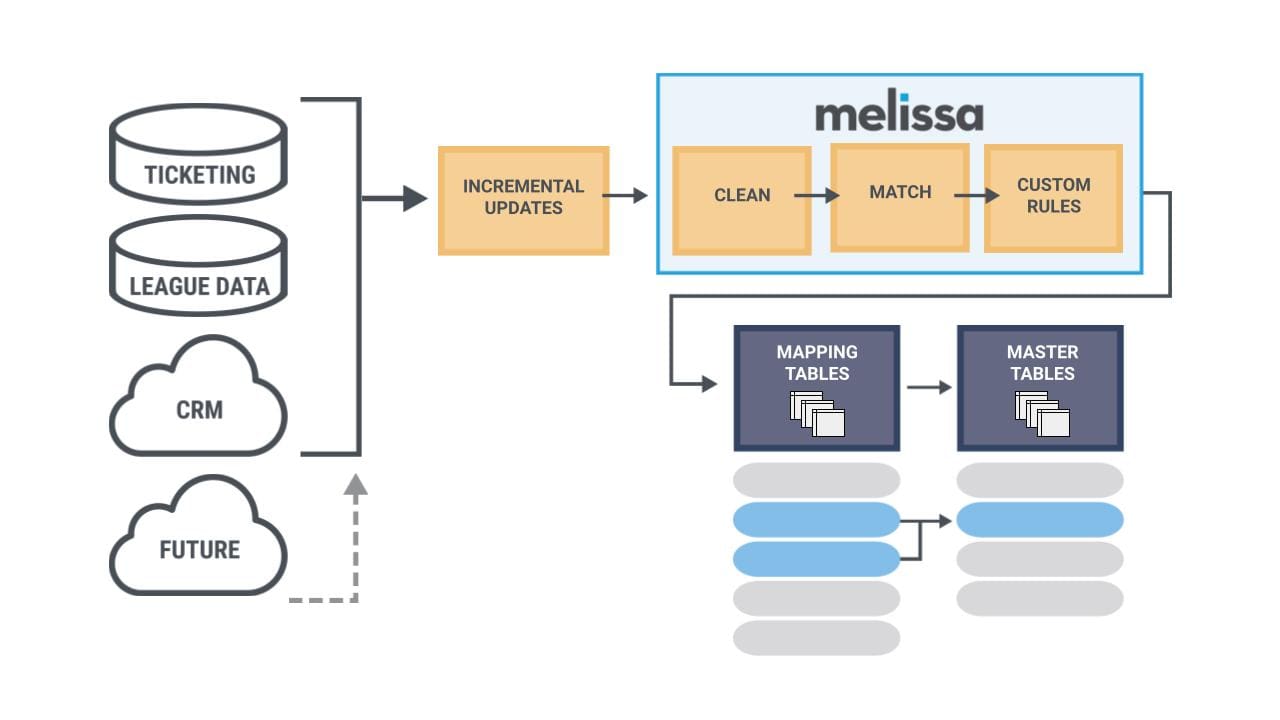
Next, the data goes through another Melissa service that applies rules that start with a fan’s first and last name as an anchor, then match across email address, mailing address, and phone number against the entire fan database of millions of records. Melissa then returns a master key for all matches.
The result? A “mapping table” that contains all the original fan contact data and the master key, providing the ability to trace back from the master key to the raw keys. This mapping table data is then rolled up into the master contact, account, and person tables.
The Giants also track historical changes to the master tables to understand what changes may have occurred over time with the master keys. In alignment with data warehousing best practices, we used a slowly changing dimension Type 2 design pattern. That means that any change to the data in a master row triggers the insertion of a new row and marks the old row as historical.